KerusCloud Use Example
Driving decision making with an adaptive clinical trial in the face of recruitment difficulties
Exploring alternative adaptive approaches to the study
KerusCloud is a revolutionary simulation-guided study design tool that ensures clinical trials are designed effectively to collect the right data, in the right patients, in the right way. Its use supports evidence-based design decisions to extensively de-risk real clinical studies, reducing development time, costs and patient burden.
The Challenge
A sponsor biopharmaceutical company were experiencing significant recruitment difficulties for an ongoing clinical trial.
The sponsor wished to explore alternative adaptive approaches to the study which would maintain integrity, especially with regards to controlling for false positive rate (alpha) but enable the possibility of stopping the study early with fewer patients than initially planned, either for efficacy or for futility.
The Approach
KerusCloud study simulation software was used to explore different group sequential designs compared to a fixed design with no interim analysis, which would allow the team to stop early for futility or efficacy. The team explored the following:
- Different stopping rules for efficacy
- Rule 1, Pocock: where the probability to stop for efficacy at the interim is higher (vs. O’Brien-Fleming). This makes it more difficult to meet success criteria at the final analysis if efficacy is not declared at the interim analysis (compared to the fixed design) i.e., “spend” more alpha at the interim.
- Rule 2, O’Brien-Fleming: where the probability to stop for efficacy at the interim is lower (vs. Pocock). This makes minimal difference to the probability of meeting success criteria at the final analysis (compared to the fixed design). i.e., “spend” less alpha at the interim.
- Whether to include a futility stopping rule or not
- Different timing of the interim analysis
- 60% through recruitment
- 75% through recruitment
- Different assumed true treatment effect
- Null
- Initially expected 10%
- Updated expected 12%
The Results
KerusCloud was used to quantify the overall probability of success (PoS) and operating characteristics (OC) under different true treatment effect size assumptions, and under different design choices (timing of interim and stopping rules) (Table 1).
These were rapidly and reliably quantified and then visualised in an interactive heatmap, which allowed both the interim and end of study results to be explored for decision-making (Figure 1). Where a treatment effect exists, the overall PoS for the fixed design was higher than for any of the adaptive designs, with the reduction of PoS being largest when there was both a futility and efficacy stopping rule at the interim (columns 4 and 5). The later the interim, the less that reduction in PoS (column 5 vs. column 4). Type 1 error is controlled at approximately 5% in each design (row 1).
Table 1. Design options explored using KerusCloud.
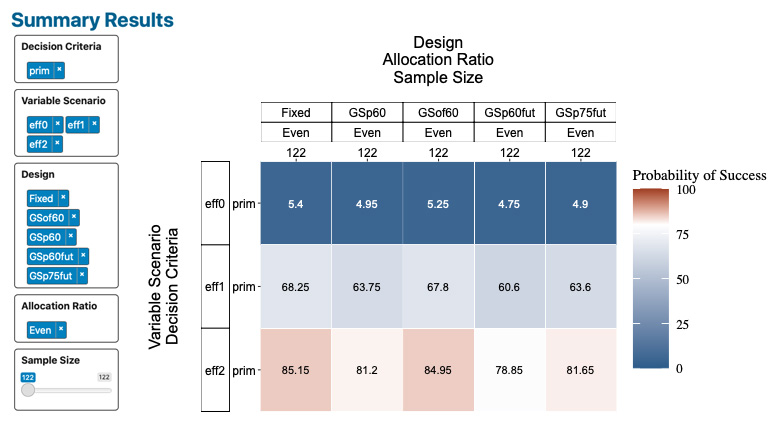
Figure 1. A KerusCloud heatmap showing the PoS values for different study scenarios, where dark blue indicates very low PoS and dark red indicates very high PoS.
Figure 2 shows the operating characteristics of a particular design, showing in this case, the trial would stop for efficacy or futility over 70% of the time at the interim stage, which was a very desirable option for the sponsor. The trade-off is the 6.3% reduction in overall probability of success vs. the fixed design.
Figure 2. Advanced characteristics of a design option from the heatmap displayed in Figure 1. The drill down graphical and tabular outputs for this design option show the number of participants included in the analysis, success/failure compared to the fixed design and any bias in the observed metric versus the fixed design that came about from examining the data earlier than planned.
The mean number of participants (across all simulations) in each design can be visualised by KerusCloud (Figure 3).
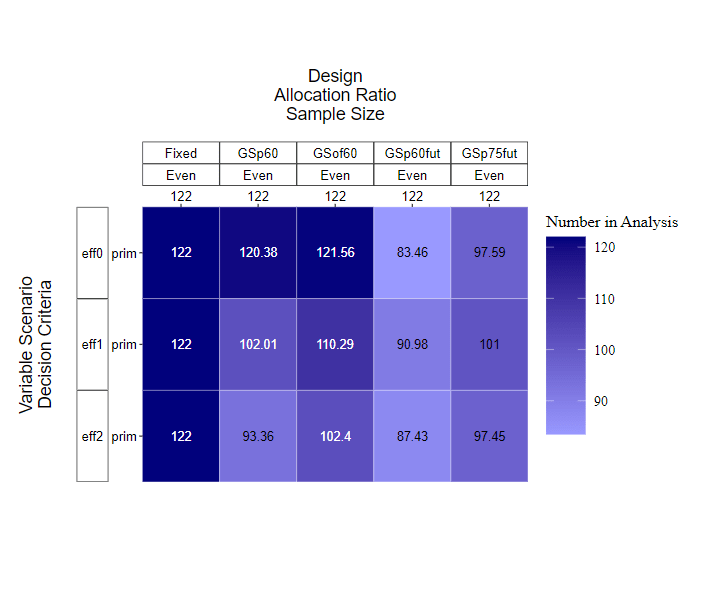
Figure 3. Mean number of participants analysed in each design type under consideration, where dark purple indicates a larger sample size and light purple indicates a smaller sample size.
Together, these results allowed a multidisciplinary team to:
- quantify and debate the merits of different design options.
- make an informed decision which also described elements in the study design which were uncertain.
- decide where they wanted to spend the “alpha” and understand the implications for the overall study in looking at the data part way through for decision-making.
The Impact
Simulation with KerusCloud provided key insights for the team when making decisions around the required sample size to support the design of this clinical trial, highlighting the benefits of simulation to fully explore the risks for a study.
- The recommendation of an interim analysis using established adaptive design methodology ensured:
- if the conclusion from the interim analysis was to continue recruiting, then the sponsor could do so with the confidence that this was necessary to obtain the appropriate PoS
- an understanding of what the most likely outcome from this interim analysis would be, so that appropriate plans could be put into place
- These insights allowed the sponsor to quantify the reduction in PoS they would incur if they decided to introduce an adaptive interim analysis. They were able to identify the adaptive design that gave them the right balance with regards to benefit (smaller average sample size) and risk (probability of making an incorrect decision at interim or final analysis).
- Unique to KerusCloud, the PoS was calculated using simulated clinical trials, which mimic real-life data as accurately as possible, going beyond theoretical sample size calculations and providing a more accurate PoS.
- Ultimately, the results provided the team with options for a de-risked study, with the potential to save time, costs and patients.
Let’s talk!
If you’d like to discuss this use case example further or learn more on how our technology enabled services can support your development project, please contact our VP of Sales & Marketing, Abbas Shivji, at abbas.shivji@exploristics.com or book a call.