Case Study
Creating Go/No Go Decision-Making Criteria for Early-Stage Studies
KerusCloud is a revolutionary simulation-guided study design tool that ensures clinical trials are designed effectively to collect the right data, in the right patients, in the right way. Its use supports evidence-based design decisions to extensively de-risk real clinical studies, reducing development time, costs and patient burden.
The Challenge
A privately held biotechnology company was developing a novel therapeutic targeting fibrosis and inflammation. Following completion of a successful first-in-human Phase I study evaluating the investigative agent in healthy adult subjects and subjects with suspected non-alcoholic steatohepatitis (NASH) and liver fibrosis, the company wanted to explore safety and efficacy of its continued administration over 12 weeks while observing changes from baseline in metabolic, inflammatory and fibrosis biomarkers.
Regulatory guidelines usually require biopsy evidence of improvement for registration of a new treatment in NASH, however, the short duration and small sample size meant that a liver biopsy was not likely to be informative. Therefore, the company sought alternative sources of evidence to inform decisions on the development of the novel NASH agent at this early stage.
The Approach
1. Creating a go/no go decision-making framework
Exploristics generated a formal go/no go decision-making quantitative framework based on a method from Lalonde et al., (1) and Frewer et al., (2). This method uses the upper and lower confidence intervals of a treatment effect to categorise the strength of evidence of the observed result into three decisions: Go; Consider; Stop. The boundary of these confidence intervals is compared to the lowest reference value (LRV) and a target value (TV), where LRV is the smallest clinically meaningful treatment effect and TV is the desired effect. These values are then used to construct a decision framework as shown in Figure 1.
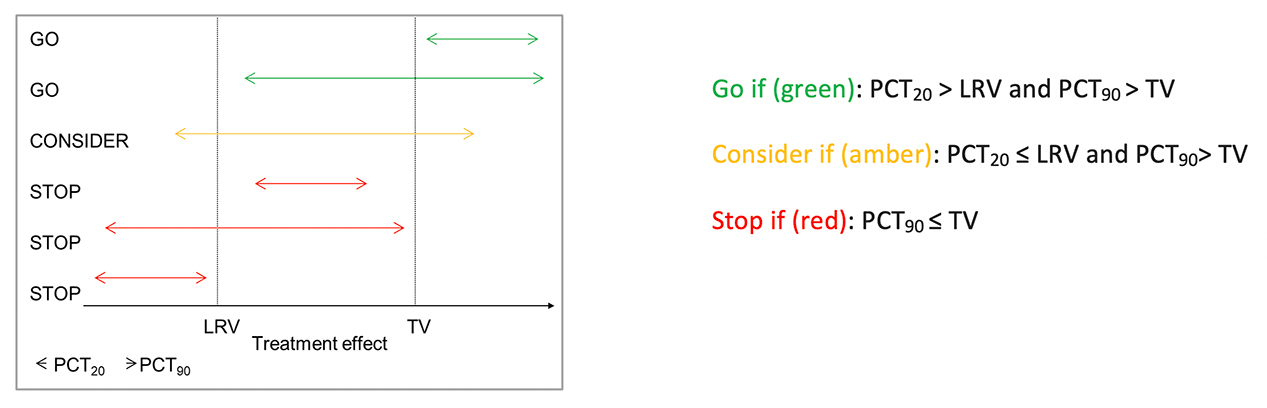
Figure 1. Visualisation of the decision framework, where PCTx denotes the xth percentile of the distribution of the treatment effect (P(∆)).
The framework provided evidence to support three possible study outcomes:
- A go decision could be made where there is ≥ 80% confidence that the true treatment effect is greater than the lower reference value (Δ>LRV).
- A stop decision could be made when there is ≤ 10% confidence that the true treatment effect is greater than the target value (Δ>TV).
- A treatment effect that does not meet the Go or Stop criteria then falls into the consider zone where more data may be required.
2. Setting Up Decision-Making Criteria using KerusCloud
To set up a decision framework for the projected study the TV and LRV needed to be defined for relevant biomarkers and the pre-specified risks set. The sample size required for the study was obtained through simulation using KerusCloud. This required:
- Key statistical information such as the statistical distributions and variation of the endpoints in the target patient population to be sourced via an extensive literature review for the metabolic, inflammatory and fibrosis biomarkers of interest.
- Construction of a decision framework using the Lalonde method once the TV, LRV, risks, sample size and variability had been determined.
- Evaluation of the framework’s performance by obtaining the probability of being in each of the three zones (go, stop, consider) under different truths of the treatment effect, using KerusCloud’s interactive heatmap (Figure 2).
- Testing the framework with 20% and 50% of the data missing to mitigate for trial site closures due to the ongoing global COVID-19 pandemic.
The Results
KerusCloud:
- Successfully incorporated the collated information into a decision-making framework to support decisions for the projected safety and efficacy study of the candidate NASH treatment.
- The framework was shown to be robust in the event of a large proportion of missing data.
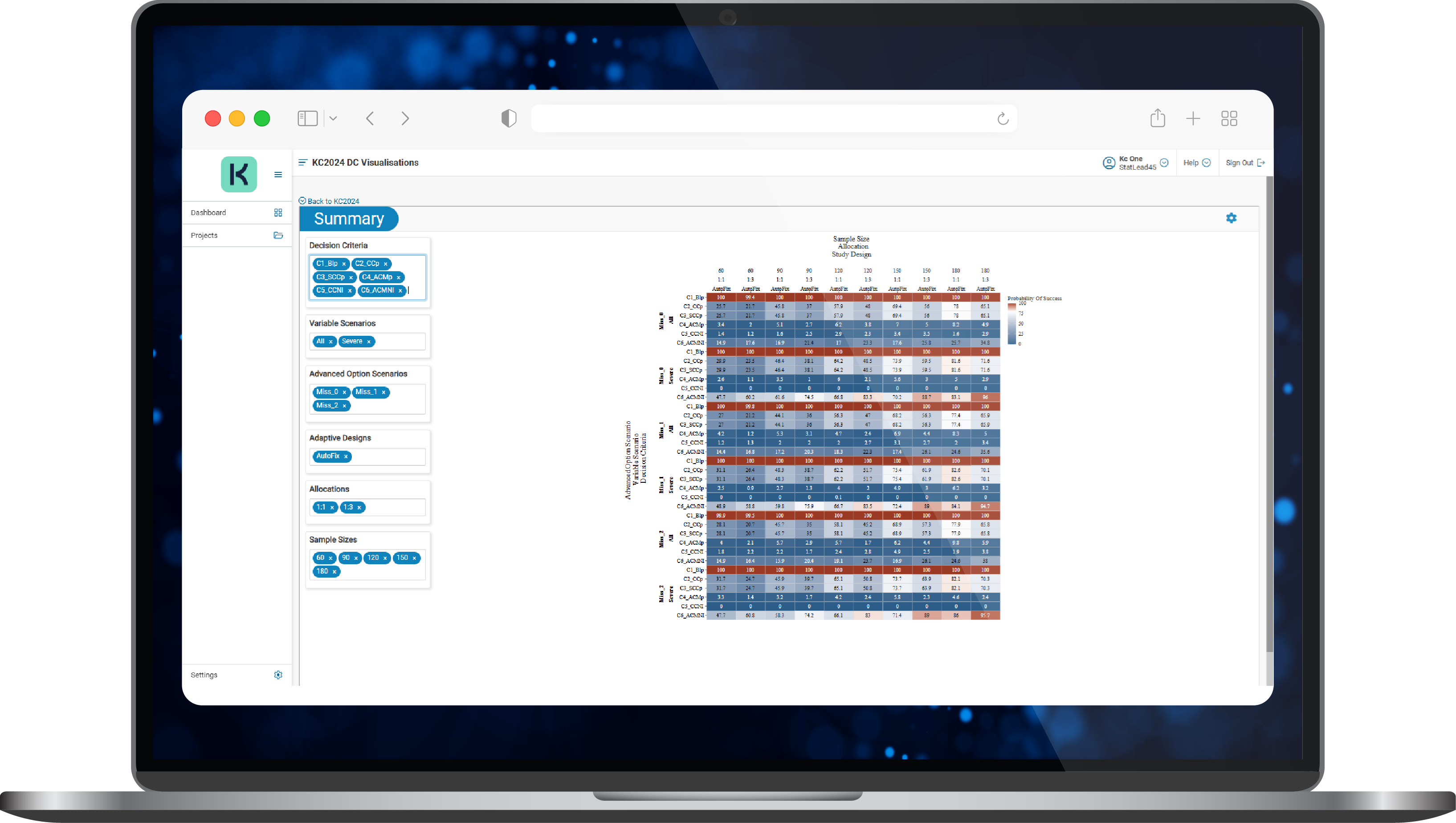
Figure 2. A typical results heatmap in KerusCloud
The Impact
Using KerusCloud:
- The client obtained a clear decision-making framework for the projected study, de-risking and accelerating the development path for their investigative agent.
- Key exploratory biomarkers for NASH were integrated into the decision framework.
- Mitigation for missing data gave the client an option to report on a smaller sample size in the event of clinical trial site closures resulting from the COVID-19 pandemic.
References
- Lalonde, R. L., Kowalski, K. G., Hutmacher, M. M., Ewy, W., Nichols, D. J., Miligan, P. A., Corrigan, B. W., Lockwood, P. A., Marshall, S. A., Benincosa, L. J., Tensfeldt, T. G., Parivar, K., Amantea, M., Glue, P., Koide, H. and Miller, R. Model-based drug development. Clinical Pharmacology and Therapeutics 2007; 82:21-32.
- Frewer, P., Mitchell, P., Watkins, C. and Matcham, J. Decision-making in early clinical drug development. Pharmaceutical Statistics 2016;15: 255-263.
Let’s talk!
If you’d like to discuss this case study further or learn more on how our technology enabled services can support your development project, please contact our VP of Sales & Marketing, Abbas Shivji, at abbas.shivji@exploristics.com or book a call.