De-risk respiratory studies upfront with access to the Pulmonary Suite for informed and optimised clinical trial design.
Patient burden, costs and development timelines can be dramatically reduced with more efficient study planning and design. Our Pulmonary Suite of data models contain accurate data related to pulmonary indications from a range of reliable sources, collated and curated by our experienced Data Science team. We use these to help you pinpoint the information you need to inform study design assumptions for your respiratory clinical trial, such as the right endpoints, biomarkers, and study population.
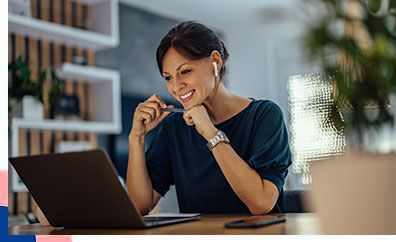
What does it offer?
The pulmonary suite provides reliable, ready-to-use historical data across a range of pulmonary indications (Figure 1). Offered at different complexity levels according to project demands, the data models give an extensive overview of the pulmonary clinical trial landscape, clinical outcomes, and patient characteristics. This information can then inform study simulations in KerusCloud to support evidence-based clinical trial design.
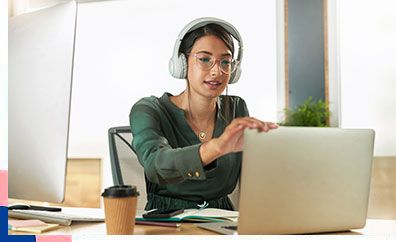
How can it be searched?
Data Science Services can sift through information relating to thousands of clinical trials in the suite, identifying relevant historical data to inform the planning of your study. The suite can be searched by:
- Disease
- Endpoint
- Patient population
- Biomarker
- Interventions
- Phase
- Enrolment
- Design
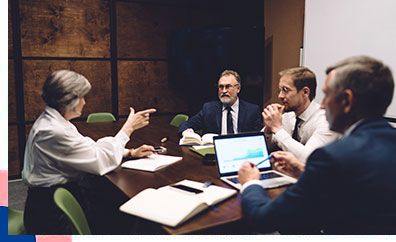
How is this useful?
Selected information can be built into realistic synthetic patient-level datasets for use in running virtual clinical trials in KerusCloud. Trial scenarios of interest can be simulated, and their outcomes examined to answer key ‘what if’ questions relating to study design and analysis. This ensures you identify, with confidence, the right approach for your pulmonary asset in a real clinical trial.
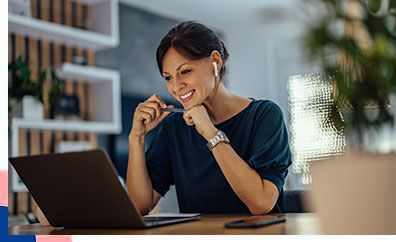
What does it offer?
The pulmonary suite provides reliable, ready-to-use historical data across a range of pulmonary indications (Figure 1). Offered at different complexity levels according to project demands, the data models give an extensive overview of the pulmonary clinical trial landscape, clinical outcomes, and patient characteristics. This information can then inform study simulations in KerusCloud to support evidence-based clinical trial design.
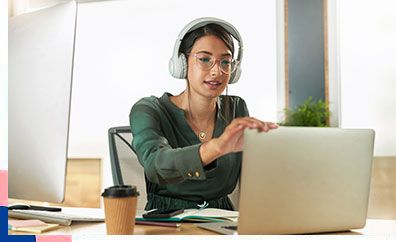
How can it be searched?
Data Science Services can quickly sift through information relating to thousands of clinical trials in the Pulmonary Suite to identify relevant historical information to inform the planning of your study. The Suite can be searched by:
- Disease
- Endpoint
- Patient population
- Biomarker
- Interventions
- Phase
- Enrolment
- Design
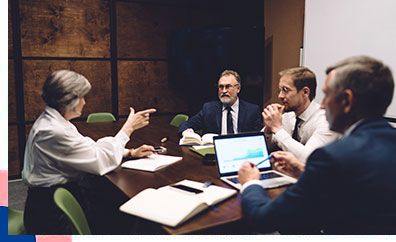
How is this useful?
Selected information can be built into realistic synthetic patient-level datasets for use in running virtual clinical trials in KerusCloud. Trial scenarios of interest can be simulated, and their outcomes examined to answer key ‘what if’ questions relating to study design and analysis. This ensures you identify, with confidence, the right approach for your pulmonary asset in a real clinical trial.
Pulmonary Suite
Complexity Level Overview